The Non-fiction Feature
Also in Bulletin #45:
The Children’s Spot: The Cartoon Guide to Statistics by Larry Gonick & Woollcott Smith
The Product Spot: Khan Academy
The Pithy Take & Who Benefits
Journalist and lecturer Charles Wheelan asks us not to be afraid: the mountain of statistics is surmountable and understanding the basics of statistics can lead to a heightened understanding of many other fields: sports, politics, business, health. He explains common statistical concepts (inference, descriptive statistics, correlation, probability, etc.) using illuminating examples while providing helpful mathematical explanations in short appendices to each chapter.
I think this book is for people who seek to understand:
(1) the underlying structure of statistics and how it functions to encapsulate and explain complex data sets;
(2) how statistical tools enable us to react appropriately to important social questions, evaluate the effectiveness of policies, and make better decisions; and
(3) how to spot those who manipulate the same tools to serve their own ends.
The Outline
The preliminaries
- Statistics–useful but dangerous–can be overly accessible in that anyone with data and a computer can do sophisticated statistical procedures in a few minutes. If the data is poor, or if the statistical techniques are used improperly, the conclusions can be recklessly misleading.
Inference
- One key function of statistics is to use the data we have to make informed conjectures about larger questions for which we do not have full information–making inferences about the unknown.
- For example, how many homeless people live in Chicago?
- It’s expensive and difficult to count the actual homeless population, but it’s important to have an estimate.
- One statistical practice is sampling, which involves gathering data for a small area and then using that to make an informed judgment about the city’s homeless population.
- For example, how many homeless people live in Chicago?
- If you read that people who eat 20 bran muffins a day have lower rates of colon cancer than people who don’t, the underlying research probably looked like this:
- In a large data set, researchers determined that people who ate at least 20 bran muffins a day had a lower incidence of colon cancer than those who did not.
- The disparity in colon cancer outcomes couldn’t be explained by just chance; so, there’s a statistically significant association between eating 20 bran muffins a day and a lower incidence of colon cancer.
- But a corresponding headline that says “20 bran muffins a day help keep colon cancer away” is misleading.
- The study never made this claim; it just showed a negative correlation between eating bran muffins and the incidence of colon cancer.
- This statistical association is not enough to prove that the bran muffins lead to better health–those who eat bran muffins may do lots of other things that lower their cancer risk, like exercising regularly.
- This also says nothing about the size of the association: how much lower is the incidence of colon cancer?
- In a large data set, researchers determined that people who ate at least 20 bran muffins a day had a lower incidence of colon cancer than those who did not.
Descriptive statistics
- A bowling score, batting average, and GPA are descriptive statistics. An overreliance on descriptive statistics can lead to misleading conclusions. Descriptive statistics exist to simplify, which implies some loss of detail.
- Descriptive statistics can be like online dating profiles: technically accurate yet pretty misleading.
- The mean, or average, can be problematic because outliers can distort it.
- Imagine ten people sitting in a bar and each earns $35,000; the mean annual income is $35,000. Bill Gates, with an annual income of $1 billion, walks in. Suddenly, the mean annual income is $91 million.
- If you said that the bar patrons had an average annual income of $91 million, that’s statistically correct but misleading.
- The mean’s sensitivity to outliers is why we shouldn’t gauge the middle class’s economic health by per capita income.
- Imagine ten people sitting in a bar and each earns $35,000; the mean annual income is $35,000. Bill Gates, with an annual income of $1 billion, walks in. Suddenly, the mean annual income is $91 million.
- The median is the point that divides a distribution in half: half the observations lie above the median and half lie below.
- The median annual income for the bar patrons was $35,000, and when Bill Gates walked in, the median annual income was still $35,000.
- The key is determining whether the mean or median is more accurate in a particular situation (a phenomenon that is easily exploited).
- The mean, or average, can be problematic because outliers can distort it.
- The benefit of these descriptive statistics is that they describe where a particular observation lies compared with everyone else.
- An “absolute” number has intrinsic meaning; if it’s 60 degrees outside, that’s an absolute figure that can be interpreted without any additional information.
- If I place ninth in a golf tournament, that’s a relative statistic.
- A relative value has meaning only in comparison to something else.
- Another statistic that helps describe jumbles of numbers is the standard deviation, which is a measure of how dispersed the data are from their mean.
- If you tracked the weights of all the people on a plane, including babies and football players, there’s a wide range of numbers, all circling a midpoint (as opposed to the weights of babies in a daycare classroom).
- The standard deviation is the descriptive statistic that assigns a number to this dispersion around the mean.
- If you tracked the weights of all the people on a plane, including babies and football players, there’s a wide range of numbers, all circling a midpoint (as opposed to the weights of babies in a daycare classroom).
- Percentage change is not the same as change in percentage points.
- Rates are often expressed in percentages. (Illinois’s sales tax rate is 6.75%.)
- The changes in rates can be described in vastly different ways.
- For example, the Illinois personal income tax increased from 3% to 5%. There are two ways to express this tax change:
- The state income tax rate increased by two percentage points.
- The state income tax increased by 67%.
- For example, the Illinois personal income tax increased from 3% to 5%. There are two ways to express this tax change:
Deceptive description
- “Precision” versus “accuracy”
- Precision reflects the exactitude with which we express something.
- If you ask someone how far away the nearest gas station is, and someone says it’s 1.265 miles to the east, that’s precise.
- If someone says, “Drive ten minutes until you see a hot dog stand and the gas station will be a couple hundred yards after that on the right,” that’s less precise but much more helpful.
- Accuracy is a measure of whether a figure is consistent with the truth.
- If an answer is accurate, then more precision is better. But no amount of precision makes up for inaccuracy.
- Precision reflects the exactitude with which we express something.
- The most common measure for school and teacher quality is test scores, but examining only test scores presents an inaccurate picture.
- There are schools with disadvantaged populations in which teachers may be doing a remarkable job but the test scores will still be low.
Correlation
- Correlation measures the degree to which two things are related to one another.
- For example, there is a correlation between summer temperatures and ice cream sales: when one goes up, the other does, too.
- Correlation can be used to encapsulate an association between two variables in a single descriptive statistic: the correlation coefficient.
- A correlation of 1 means that every change in one variable is associated with an equal change in the other variable in the same direction.
- A correlation of -1 means that every change in one variable is associated with an equal change in the other in the opposite direction.
- A correlation of 0 means that the variables have no meaningful association (like the relationship between shoe size and SAT scores).
Probability
- Probability is the study of events and outcomes that involve uncertainty.
- If you flip a coin four times in a row, you can’t know the outcome in advance with certainty, but you can determine that some outcomes (two heads, two tails) are more likely than others (four heads).
- Some events have probabilities that can be inferred on the basis of past data.
- The probability of kicking the extra point after a touchdown in professional football is 0.94.
- The entire insurance industry is built on probability.
- When you insure anything, you’re contracting to receive a specified payoff in the event of clearly defined contingency.
- As a consumer, insurance will not save money in the long run, but it will prevent an unacceptably high loss (like a house that has burned down).
Problems with probability
- Assuming events are independent when they are not.
- Assume you run risk management at a major airline. The probability of a jet engine failing during a flight is 1 in 100,000. Each jet has two engines. Your assistant assumes that the risk of both engines shutting down is 1/100,000 squared, or 1 in 10 billion.
- But the two engine failures are not independent events. If a plane flies through a flock of geese, or there are maintenance issues, both engines are likely compromised.
- If one engine fails, the probability of the second engine failing is significantly higher than 1 in 100,000.
- But the two engine failures are not independent events. If a plane flies through a flock of geese, or there are maintenance issues, both engines are likely compromised.
- Assume you run risk management at a major airline. The probability of a jet engine failing during a flight is 1 in 100,000. Each jet has two engines. Your assistant assumes that the risk of both engines shutting down is 1/100,000 squared, or 1 in 10 billion.
- Not understanding when events are independent.
- If the roulette ball has landed on black five times in a row, the chances of it landing on red have not increased–it remains unchanged: 16/38.
- The prosecutor’s fallacy
- Suppose a prosecutor tells a jury that the DNA sample found at the crime scene matches the defendant’s sample, and there’s only a one in a million chance that the sample would match anyone but the defendant’s.
- It’s possible that, for whatever reason, the defendant’s DNA was previously included in a national DNA database of millions of felons.
- The chances of finding a one in a million match are relatively high in a database with samples from a million people.
- Suppose a prosecutor tells a jury that the DNA sample found at the crime scene matches the defendant’s sample, and there’s only a one in a million chance that the sample would match anyone but the defendant’s.
- Reversion to the mean
- Probability tells us that any outlier is likely to be followed by outcomes that are more consistent with the long-term average.
- When a team is featured on the cover of Sports Illustrated, they usually see their performance fall off afterwards.
- It’s likely that the team appeared on the cover after a good stretch and their subsequent performance merely reverts to normal.
- When a team is featured on the cover of Sports Illustrated, they usually see their performance fall off afterwards.
- Probability tells us that any outlier is likely to be followed by outcomes that are more consistent with the long-term average.
- Statistical discrimination
- If we can build a statistical model that correctly identifies drug smugglers 80/100 times, what happens to the 20% of people the model incorrectly identifies? They will be harassed over and over again.
Polls
- Polling is more than just statistical inference–it’s an inference about the opinions of a certain population, based on the views expressed by a sample of that population.
- According to Gallup, in each year since 2002, over 60% of Americans said that they favor the death penalty. But, support for the death penalty plummets when life imprisonment without parole is offered as an alternative.
- When soliciting public opinion, the phrasing of the question and the choice of language matters enormously.
- Politicians often exploit this phenomenon by using polls and focus groups to test words that work.
- According to Gallup, in each year since 2002, over 60% of Americans said that they favor the death penalty. But, support for the death penalty plummets when life imprisonment without parole is offered as an alternative.
Regression analysis
- The data present unorganized clues, and statistical analysis is the detective work that crafts the raw data into meaningful conclusions.
- Researchers use regression analysis to isolate a relationship between two variables, such as smoking and cancer, while holding constant the effects of other variables, such as diet, exercise, weight, and so on.
- Then, researchers quantify the association between smoking and the increased rate of lung cancer.
- Another example addresses whether stress on the job can kill you.
- CEOs are at significantly less risk than their secretaries.
- The most dangerous kind of job stress stems from having low control over one’s responsibilities.
- Researchers collected detailed longitudinal data on thousands of British civil service employees and compared their health outcomes.
- Researchers used regression analysis to quantify the relationship between a particular variable and the outcome that we care about.
- CEOs are at significantly less risk than their secretaries.
- Researchers use regression analysis to isolate a relationship between two variables, such as smoking and cancer, while holding constant the effects of other variables, such as diet, exercise, weight, and so on.
- Most of the studies in the news are based on regression analysis.
Program evaluation
- Program evaluation measures the causal effect of some intervention, like a new cancer drug or a job placement program for high school dropouts.
- The challenge with “before and after” analyses is that just because one thing follows another doesn’t mean that there’s a causal relationship.
- First, researchers examine the “before and after” data for the treatment group, such as the unemployment figures for a country that has implemented a job training program.
- Second, they compare those data with the unemployment figures over the same time period for a similar country that didn’t implement any such program.
And More, Including:
- One of the most irresponsible uses of statistics in recent memory: the mechanism for gauging risk on Wall Street prior to the 2008 financial crisis
- A brief, statistical evaluation of: what (if anything) is causing the rise in the incidence of autism; how can we identify and reward good teachers and schools; what the best tools are for fighting global poverty
- A short summary of the best available statistical software, such as Microsoft Excel and IBM SPSS
- The vital importance of good data–without good data, there are no good statistics
- The central limit theorem and its critical functions
- Common mistakes with regression analysis
- A Bernoulli trial with Schlitz (beer) and Michelob (beer)
Naked Statistics: Stripping the Dread from the Data
Author: Charles Wheelan
Publisher: W.W. Norton & Company
304 pages | 2014
Purchase
[If you purchase anything from Bookshop via this link, I get a small percentage at no cost to you.]
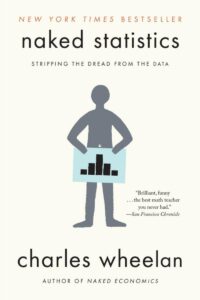